- Home
- Speech Synthesis
- What is Word Error Rate (WER)?
What is Word Error Rate (WER)?
Looking for our Text to Speech Reader?
Featured In
In the world of natural language processing and automatic speech recognition (ASR), measuring the accuracy of speech-to-text systems is crucial. One common metric used for this purpose is the Word Error Rate (WER), which provides insights into how effectively a system converts spoken language into text. This metric is pivotal in developing and refining ASR technologies by companies like Microsoft, IBM, and Amazon, which are at the forefront of innovations in speech recognition systems.
Understanding WER
WER is a metric derived from the Levenshtein distance, an algorithm used to measure the difference between two sequences. In the context of ASR, these sequences are the transcription produced by the speech recognition system (the "hypothesis") and the actual text that was spoken (the "reference" or "ground truth").
The computation of WER involves counting the number of insertions, deletions, and substitutions required to transform the hypothesis into the reference transcript. The formula for WER is given by:
\[ \text{WER} = \frac{\text{Number of Substitutions} + \text{Number of Deletions} + \text{Number of Insertions}}{\text{Total Number of Words in the Reference Transcript}} \]
Significance in Real-World Applications
WER is especially important in real-time, real-world applications where speech recognition systems must perform under various conditions, including background noise and different accents. A lower WER indicates a more accurate transcription, reflecting a system's ability to understand spoken language effectively.
Factors Influencing WER
Several factors can affect the WER of an ASR system. These include the linguistic complexity of the language, the presence of technical jargon or uncommon nouns, and the clarity of the speech input. Background noise and the quality of the audio input also play significant roles. For instance, ASR systems trained on datasets with diverse accents and speaking styles are generally more robust and yield a lower WER.
The Role of Deep Learning and Neural Networks
The advent of deep learning and neural networks has significantly advanced the field of ASR. Generative models and large language models (LLMs), which leverage vast amounts of training data, have improved the understanding of complex language patterns and enhanced transcription accuracy. These advancements are integral to developing ASR systems that are not only accurate but also adaptable to different languages and dialects.
Practical Use Cases and ASR System Evaluation
ASR systems are evaluated using WER to ensure they meet the specific needs of various use cases, from voice-activated assistants to automated customer service solutions. For example, an ASR system used in a noisy factory environment will likely focus on achieving a lower WER with robust noise normalization techniques. Conversely, a system designed for a lecture transcription service would prioritize linguistic accuracy and the ability to handle diverse topics and vocabulary.
Companies often utilize WER as part of their quality assurance for speech recognition products. By analyzing the types of errors—whether they are deletions, substitutions, or insertions—developers can pinpoint specific areas for improvement. For instance, a high number of substitutions might indicate that the system struggles with certain phonetic or linguistic nuances, while insertions could suggest issues with the system's handling of speech pauses or overlapping talk.
Continuous Development and Challenges
The quest to lower WER is ongoing, as it involves continuous improvements in machine learning algorithms, better training datasets, and more sophisticated normalization techniques. Real-world deployment often presents new challenges that were not fully anticipated during the system's initial training phase, necessitating ongoing adjustments and learning.
Future Directions
Looking forward, the integration of ASR with other aspects of artificial intelligence, such as natural language understanding and context-aware computing, promises to enhance the practical effectiveness of speech recognition systems further. Innovations in neural network architectures and the increased use of generative and discriminative models in training are also expected to drive advancements in ASR technology.
Word Error Rate is a vital metric for assessing the performance of automatic speech recognition systems. It serves as a benchmark that reflects how well a system understands and transcribes spoken language into written text. As technology evolves and more sophisticated tools become available, the potential to achieve even lower WERs and more nuanced language understanding continues to grow, shaping the future of how we interact with machines.
Frequently Asked Questions
The word error rate (WER) is a metric used to evaluate the accuracy of an automatic speech recognition system by comparing the transcribed text to the original spoken text.
A good WER varies by application, but generally, lower rates (closer to 0%) indicate better transcription accuracy, with rates below 10% often seen as high-quality.
In text, WER stands for Word Error Rate, which measures the percentage of errors in a speech recognition system's transcription compared to the original speech.
CER (Character Error Rate) measures the number of character-level errors in a transcription, while WER (Word Error Rate) measures the number of word-level errors.
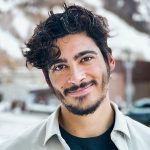
Cliff Weitzman
Cliff Weitzman is a dyslexia advocate and the CEO and founder of Speechify, the #1 text-to-speech app in the world, totaling over 100,000 5-star reviews and ranking first place in the App Store for the News & Magazines category. In 2017, Weitzman was named to the Forbes 30 under 30 list for his work making the internet more accessible to people with learning disabilities. Cliff Weitzman has been featured in EdSurge, Inc., PC Mag, Entrepreneur, Mashable, among other leading outlets.